The Data Challenge: Why Measurement Matters Now More Than Ever
Did you know that 78% of marketers struggled with accurate attribution in 2024? With the deprecation of third-party cookies, the rise of AI-powered analytics, and increasingly sophisticated customer journeys, we’re seeing a revolutionary shift in how we track and measure marketing success.
Let me walk you through what’s working in 2025 and how you can stay ahead of the curve.
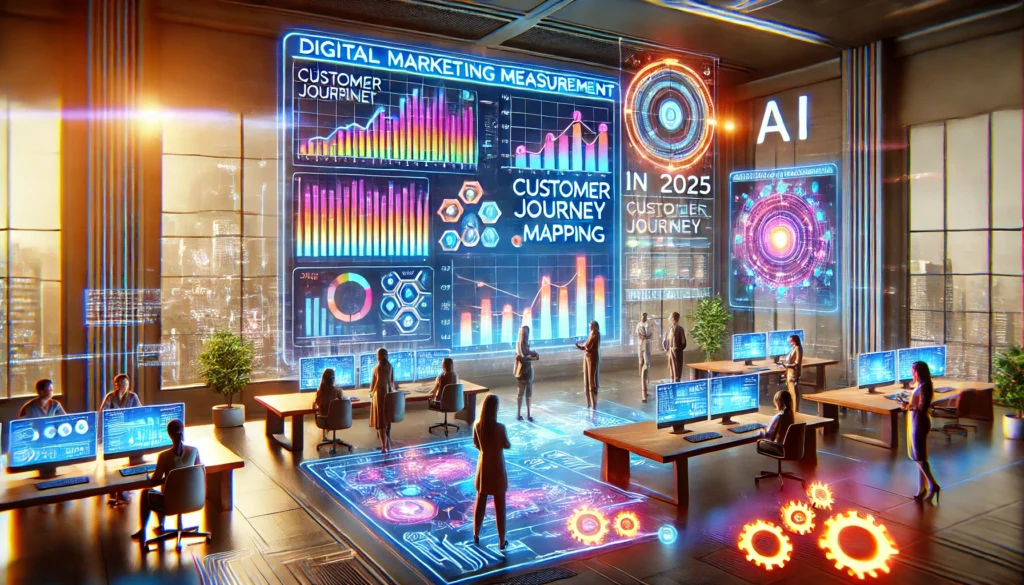
When Privacy Regulations Changed Everything
Let me tell you something that still keeps me up at night: the day Google first announced they were deprecating third-party cookies. I was managing analytics for several clients, and I distinctly remember thinking, “Well, there goes our entire measurement strategy!”
But that chaos led to one of the most innovative periods in digital marketing measurement.
Server-Side Tracking: Your New Best Friend
Server-side tracking has become absolutely crucial in our privacy-first world. The data accuracy can be significantly improved due to reduced ad blocker interference.
The key is setting up proper data streams and ensuring your server-side tagging is configured correctly.
The Power of Digital Vaults: Data Clean Rooms
Think of data clean rooms as secure digital vaults where you can analyze user-level data without actually accessing personal information.
Tools like Google Ads Data Hub and Amazon Marketing Cloud are game-changers for measuring campaign effectiveness while maintaining user privacy.
Pro tip: Start with smaller, focused analyses in your clean room before attempting complex cross-channel attribution projects.
First-Party Data: The Real MVP
I can’t stress this enough, if you haven’t built a robust first-party data strategy yet, drop everything and start now!
We’ve had incredible success implementing progressive profiling on client websites, where we gradually collect more user information through micro-interactions rather than lengthy forms.
What is Progressive Profiling?
Progressive profiling collects user information gradually over multiple interactions, rather than asking for everything at once. It’s like getting to know someone naturally.
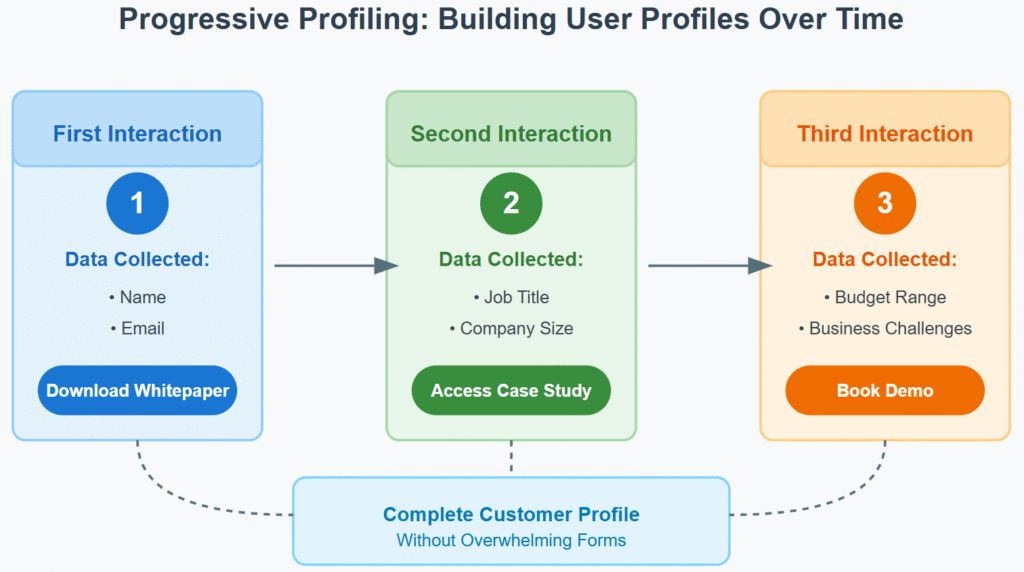
Zero-Party Data: The Trust Revolution
Zero-party data has been another revelation. This data is directly provided by customers and doesn’t rely on third-party sources.
Interactive polls and preference centers allow users to explicitly tell you what they’re interested in. It’s amazing how willing people are to share information when you’re transparent about how you’ll use it!
Building Trust Through Transparency
Consent management isn’t just about compliance; it’s about building trust. Being upfront about data collection and giving users granular control actually increases opt-in rates.
Studies show that transparent data collection methods that explain exactly how the company uses data saw a 27% higher opt-in rate than standard boring popups.
The Compliance Imperative
Privacy regulations keep evolving, and what’s compliant today might not be tomorrow. Consider developing a monthly audit checklist covering everything from checking for accidentally collected PII to verifying that your consent strings are properly passing to all your marketing tools.
The Bottom Line on Privacy-First Analytics
Privacy-first analytics isn’t about finding workarounds for cookie limitations; it’s about fundamentally rethinking measurement.
Focus on building direct relationships with your audience, be transparent about data collection, and invest in technologies that respect user privacy while still providing valuable insights.
The future of measurement isn’t about tracking everything; it’s about tracking the right things in the right way.
AI and Machine Learning: The New Measurement Powerhouse
Platform-Specific Modeling: Filling the Data Gaps
The integration of AI and machine learning into marketing measurement has become increasingly important, especially with platform-specific modeling tools.
Meta’s conversion modeling has been particularly useful since iOS 14’s privacy changes, using machine learning to estimate conversions we can’t directly track. While these modeled numbers initially seemed suspect, comparing them against actual business results has shown they’re generally reliable for decision-making.
Google’s modeling approaches in Google Analytics 4 have proven valuable for understanding cross-device journeys and filling in data gaps when we can’t track every touchpoint.
Predictive Analytics: Seeing the Future
Predictive analytics represents another key application of AI in marketing measurement. These systems analyze historical marketing data to forecast future trends and identify potential opportunities before they occur, particularly valuable for budget planning and campaign optimization.
Automated Anomaly Detection: Your 24/7 Data Guardian
Automated anomaly detection systems continuously monitor marketing data streams to identify unusual patterns that might indicate tracking problems or unexpected performance changes. This capability ensures data quality and enables faster response to technical issues.
Human + Machine: The Perfect Partnership
One thing that’s become clear: platform-specific AI tools aren’t a replacement for human expertise. They’re powerful aids that handle data processing and pattern recognition, allowing us to focus more on strategy and interpretation.
The most effective approaches combine AI capabilities with human insight and business understanding.
Keys to Success with AI-Powered Measurement
The key to success with AI-powered measurement lies in having clean, reliable first-party data to validate modeled results, plus clear objectives for what you’re measuring. Without these foundations, even the most sophisticated AI tools won’t provide meaningful insights.
Looking ahead, platform-specific modeling will become even more important as privacy regulations evolve. Understanding how to work with and validate these models will be a crucial skill for digital marketers.
Modern Attribution Methods: Solving the Cross-Channel Puzzle
The Evolution of Multi-Touch Attribution
The cross-channel attribution landscape has changed dramatically with privacy regulations and the decline of third-party cookies.
While we used to rely heavily on cookie-based user journey tracking, we now need to combine multiple approaches. Google Analytics 4’s data-driven attribution shows how machine learning can identify patterns in complex customer journeys, even with limited data.
Similarly, Meta’s attribution models have adapted to work within privacy constraints while still providing valuable insights about campaign performance.
Bridging Online and Offline: The Complete Picture
The integration of online and offline data has become more sophisticated. Using tools like Google Ads’ enhanced conversions and Meta’s Conversions API, businesses can connect their CRM data with advertising platforms in a privacy-compliant way.
This approach is crucial for businesses with significant offline conversions or long sales cycles.
Media Mix Modeling: The Comeback Kid
Media mix modeling (MMM) has made a comeback in the privacy-first era. While not new, it’s increasingly relevant as a complement to user-level attribution.
According to Deloitte research, C-Level leaders who prioritized MMM were over twice as likely to exceed their revenue goals by 10% or more.
Google’s Meridian, an open-source MMM solution, is democratizing access to sophisticated measurement techniques. It stands out for its ability to calibrate with incrementality experiments and incorporate reach and frequency data.
The Democratization of Advanced Measurement
About 60% of US advertisers are now using MMMs, and 58% of those not currently using them are considering implementation. These models help understand the broader impact of marketing efforts, including factors like seasonality and external events that user-level attribution might miss.
Incrementality Testing: Finding True Value
Incrementality testing has become a crucial part of our attribution toolkit. Through geo-experiments in Google and holdout tests in Meta, I’ve learned that attributed conversions don’t always tell the full story.
Sometimes campaigns that look great in last-click attribution actually provide minimal incremental value, and vice versa.
The Art of Measurement Triangulation
The key to successful cross-channel attribution in 2025 is understanding that no single method is perfect. The best approach combines different measurement techniques, each providing a different piece of the puzzle.
By triangulating between different data sources and measurement approaches, we can build a more accurate picture of marketing performance.
What’s most important is aligning your attribution approach with your business objectives and understanding the limitations of each method.
Modern Marketing KPIs: Metrics That Drive Business Growth
Beyond Vanity Metrics
The landscape of marketing KPIs has shifted dramatically as our understanding of meaningful metrics has evolved. Traditional metrics like click-through rates and basic conversion rates tell an increasingly small part of the story.
Today’s focus is on metrics that directly tie to business impact.
The Value-Focused Framework
Revenue Per User (RPU) and Customer Lifetime Value (CLV) have become central to modern reporting frameworks. With rising acquisition costs across most channels, understanding the long-term revenue potential of different customer segments is crucial.
Rather than reporting on cost per acquisition in isolation, focus on Customer Acquisition Cost (CAC) as a ratio of CLV.
Quality Over Quantity in Engagement
Engagement quality metrics have evolved significantly. Instead of just tracking time on site or bounce rates, we’re now measuring specific user behaviors that correlate with business outcomes.
Tracking micro-conversions like saved items, content downloads, or calculator usage often provides better insight into user intent than traditional engagement metrics.
The Retention Revolution
Customer retention and loyalty metrics have taken center stage in our privacy-first world. As acquisition becomes more challenging, metrics like repeat purchase rate, subscription retention, and customer satisfaction scores have become crucial KPIs.
The ability to track and improve these metrics relies heavily on first-party data, making them particularly valuable in our current measurement landscape.
The ROI Evolution
ROI calculation has also evolved. With multi-touch attribution challenges, we’re increasingly looking at incremental ROI through controlled experiments rather than relying solely on attributed revenue.
This means setting up proper test and control groups and measuring the true incremental impact of marketing efforts.
Quality Over Volume
One of the biggest shifts is the increased emphasis on quality metrics over volume metrics. Rather than celebrating high numbers of leads or conversions, we’re focusing more on metrics that indicate quality, like sales qualified lead (SQL) conversion rates and average order value trends.
Select KPIs that align with your specific business objectives and understand the limitations of each metric. The trend toward measuring meaningful business impact rather than vanity metrics is here to stay.
The Modern Measurement Tech Stack: Tools That Drive Insights
Foundation: Analytics Platforms
Google Analytics 4 has become the foundation of many measurement strategies. While the transition from Universal Analytics was painful, GA4’s event-based data model and built-in machine learning capabilities have proven valuable for understanding user behavior across platforms.
The direct integration with Google Ads and BigQuery makes it easier to combine and analyze data from multiple sources.
Customer Data Platforms: The Single Source of Truth
Customer Data Platforms (CDPs) like Segment and Tealium have become crucial for managing first-party data effectively. They help unify customer data across touchpoints while maintaining privacy compliance.
Their key advantage is creating persistent user profiles even as traditional tracking methods become less reliable.
Visualization and Reporting: Making Data Accessible
For reporting and visualization, tools like Looker and Tableau remain industry standards, but Looker Studio (formerly Data Studio) has become increasingly capable, especially for organizations heavily invested in the Google stack.
The ability to blend data from multiple sources while maintaining live connections to platforms like GA4 makes it a go-to for many clients.
Integration: The Critical Connector
Integration is perhaps the most critical aspect of modern measurement tech stacks. APIs and server-side tracking implementations have become essential for maintaining accurate data flow between platforms.
Server-side Google Tag Manager has been particularly valuable for improving data accuracy and reducing reliance on client-side tracking.
Data Warehouses: The Analysis Powerhouse
Data warehouse solutions like BigQuery or Snowflake have become nearly essential for serious analytics work. The ability to store and analyze large amounts of raw data, combine multiple data sources, and perform complex analyses has made them a crucial part of advanced measurement setups.
Automation: Beyond Simple Reports
Automated reporting has evolved from simple scheduled exports to sophisticated systems that can detect anomalies and provide intelligent insights.
However, the key to successful automation isn’t just setting up the tools, it’s ensuring the underlying data structure and tracking implementation are rock solid.
The Tool Selection Philosophy
The most important lesson about measurement technology is that more tools aren’t always better. Focus on selecting and properly implementing tools that align with your measurement strategy and business objectives.
It’s better to have a few well-integrated tools than a complex stack that no one fully understands how to use effectively.
The Future of Marketing Measurement
The evolution of digital marketing measurement won’t stop, but with the right approach and tools, marketers can achieve unprecedented insights into their campaigns’ performance.
The key is adapting to privacy-first measurement while leveraging AI and machine learning to fill in the gaps. Remember, successful measurement in 2025 isn’t just about collecting data, it’s about turning that data into actionable insights that drive business growth.
Iām an SEO and performance marketing leader who loves breaking down complex strategies into clear, actionable insights. I have driven growth for reputable brands such as SAP, Four Seasons, BioMarin Pharmaceutical, and Rosewood Hotels in SEO and Performance Marketing strategy.